Knowledge Generation in Visual Analytics
DFG Project in the field of "Knowledge Generation in Visual Analytics" (May 2017 until 2020)
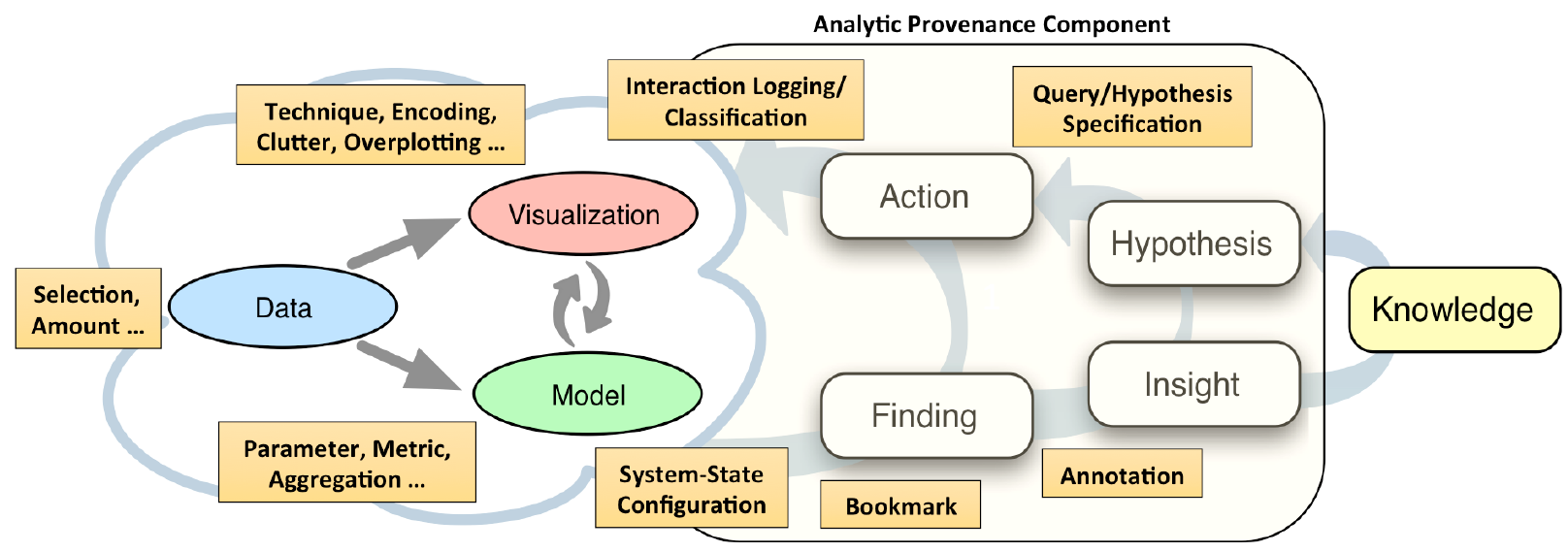
Visual Analytics (VA) combines human and machine capabilities in order to generate knowledge from data. Systems are capable of processing large amounts of data while humans reason about their problems by leveraging their knowledge. Humans participate in the VA process by steering the analysis process through interactions with the system.
Most current approaches have two major drawbacks:
- on the one hand, analysts cannot externalize their expert knowledge during the analysis process;
- on the other hand, they do not understand the processes happening in the system.
The project aims at bringing human and machine closer together in order to enhance VA for a more effective and efficient data analysis.
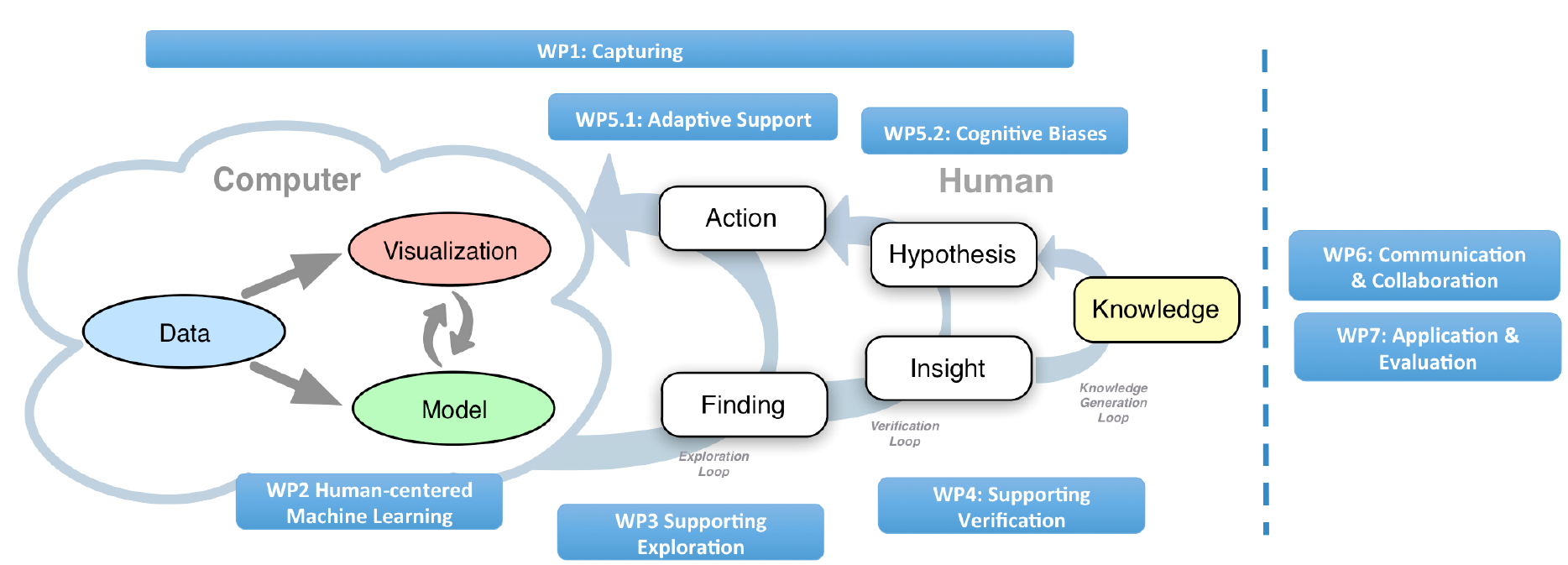
This will be achieved by bridging data and analytic provenance with automated and visualization methods. The two types of provenance information will be used to support and automate human knowledge generation processes adaptively according to the users’ needs in their current analysis stage. A major part of this research proposal is to bridge the gap between human and machine learning (ML) in order to make complex model configuration and interaction more accessible and usable.
Further, we aim to mitigate human errors during analysis processes caused by cognitive biases. The machine can be used as an unbiased counter party. The unique feature of this research proposal is a holistic perspective onto the entire knowledge generation process in VA with the goal of enhancing the state of the art by developing methods along the entire VA-pipeline.
The investigated methods will be applied to several real world datasets, domains, tasks, and users in the analysis areas of flight trajectories, political debates, and subspace clustering in high-dimensional data. The benefits of this research will be evaluated through user studies illustrating the benefits of the novel methods, which enhance the data analysis process to be more accessible, effective, efficient, transparent, and reliable.